
On ML50, we demonstrate that multilingual finetuning improves on average 1 BLEU over the strongest baselines (being either multilingual from scratch or bilingual finetuning) while improving 9.3 BLEU on average over bilingual baselines from scratch.
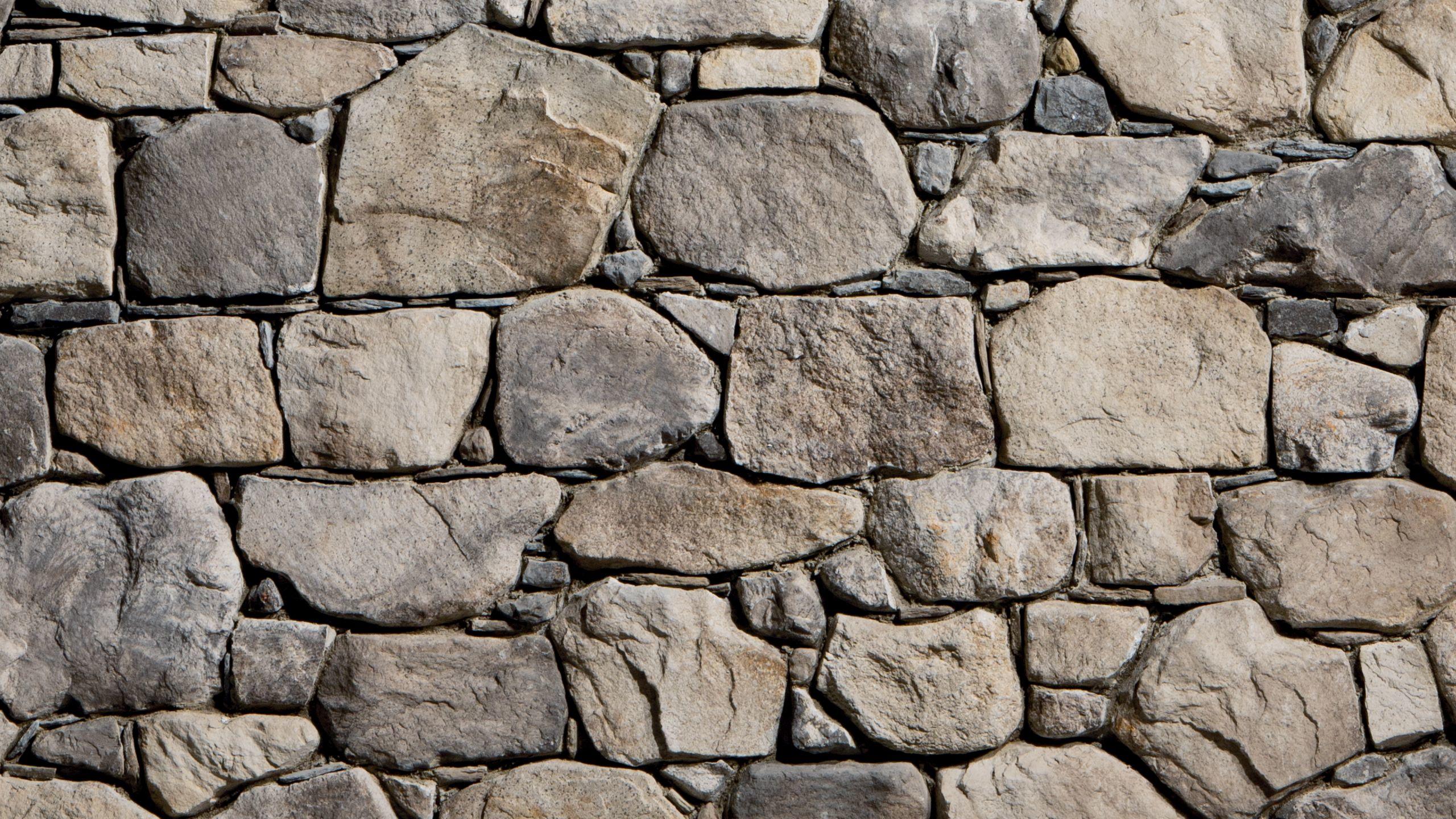
Following the approach of ParaNMT, we train a Czech-English neural machine translation (NMT. Abstract: We present ParaBank, a large-scale English paraphrase dataset that surpasses prior work in both quantity and quality. Finally, we create the ML50 benchmark, covering low, mid, and high resource languages, to facilitate reproducible research by standardizing training and evaluation data. ParaBank: Monolingual Bitext Generation and Sentential Paraphrasing via Lexically-constrained Neural Machine Translation. The rules are as follows: Remove sentences with more than 50 punc- tuation. Since it is important to clean data strictly (Wang et al.,2018), we follow m2m-100 data preprocessing procedures3to lter bitext data. It also provides tools for processing parallel and monolingual data as well as several. We double the number of languages in mBART to support multilingual machine translation models of 50 languages. We use FLORES-101 SentencePiece (SPM)1tok- enizer model with 256K tokens to tokenize bitext and monolingual sentences2. OPUS is a growing resource of freely accessible parallel corpora. Instead of finetuning on one direction, a pretrained model is finetuned on many directions at the same time. In this work, we show that multilingual translation models can be created through multilingual finetuning. We demonstrate that pretrained models can be extended to incorporate additional languages without loss of performance. Previous work in multilingual pretraining has demonstrated that machine translation systems can be created by finetuning on bitext. Compared to multilingual models trained from scratch, starting from pretrained models incorporates the benefits of large quantities of unlabeled monolingual data, which is particularly important for low resource languages where bitext is not available. This work proposes a multi-task learning (MTL) framework that jointly trains the model with the translation task on bitext data and two denoising tasks on the monolingual data, and shows the effectiveness of MTL over pre-training approaches for both NMT and cross-lingual transfer learning NLU tasks. Instead of finetuning on one direction, a pretrained model is finetuned on many directions at the same time. Language engineers: training data for statistical/neural NLP applications (e.g. Because of over-166 lap between the English portions of these two 167 bitexts, we have an implicit FRA-HAT bitext of 168 length 77,121. Previous work in multilingual pretraining has demonstrated that machine translation systems can be created by finetuning on bitext. These 163 data come from broadcasts and literature produced 164.

Recent work demonstrates the potential of multilingual pretraining of creating one model that can be used for various tasks in different languages.
